- Data Pragmatist
- Posts
- Accelerating AI Solutions with Low-Code ML Platforms
Accelerating AI Solutions with Low-Code ML Platforms
OpenAI joins Silicon Valley companies lobbying against California's AI bill
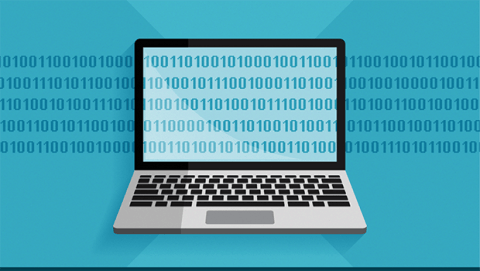

Welcome to learning edition of the Data Pragmatist, your dose of all things data science and AI.
📖 Estimated Reading Time: 5 minutes. Missed our previous editions?
👀 Elon Musk forced to reveal X investors' list LINK
A court ordered X Holdings, owned by Elon Musk, to unseal its list of shareholders, revealing notable investors like Andreessen Horowitz, Sequoia, Saudi Prince Alwaleed, Diddy, and Jack Dorsey.
The unsealing was prompted by a motion from a journalism nonprofit, arguing public interest in knowing the investors behind the influential social-media platform.
The disclosure follows X's legal battles and financial challenges, including a significant drop in advertising revenue since Musk's takeover of the platform.
⚖️ OpenAI joins Silicon Valley companies lobbying against California's AI bill LINK
OpenAI's chief strategy officer Jason Kwon argues that AI regulations should be managed by the federal government, not individual states, to avoid hindering progress and causing businesses to relocate from California.
Kwon states that a consistent, nation-wide set of AI policies will promote innovation, allowing the U.S. to become a leader in global AI standards, and thus opposes California's SB 1047 bill.
The proposed California AI safety bill, designed by Senator Scott Wiener, includes measures like pre-deployment safety testing and whistleblower protections, and awaits its final vote before potentially being signed by Governor Gavin Newsom.
Want SOC 2 compliance without the Security Theater?
Question 🤔 does your SOC 2 program feel like Security Theater? Just checking pointless boxes, not actually building security?
In an industry filled with security theater vendors, Oneleet is the only security-first compliance platform that provides an “all in one” solution for SOC 2.
We’ll build you a real-world Security Program, perform the Penetration Test, integrate with a 3rd Party Auditor, and provide the Compliance Software … all within one platform.
🧠 Accelerating AI Solutions with Low-Code ML Platforms
Organizations are increasingly turning to data and analytics to address business challenges, with 91% of enterprises investing in AI. However, only 26% of these firms have AI in widespread production due to challenges like long development cycles, model drift, and talent shortages. Low-code machine learning (ML) platforms offer a solution by streamlining the development process and addressing these key obstacles.
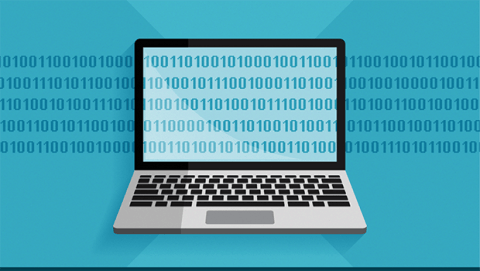
Challenges in AI Implementation
AI projects often face long cycle times, with 80% of companies taking six months to productionize a model. Additionally, model drift, where models lose accuracy due to changes in data or business dynamics, poses a significant risk. Furthermore, there is a shortage of skilled data scientists, making it difficult for organizations to build and maintain AI models.
Benefits of Low-Code ML Platforms
Low-code platforms address these challenges by providing a visual, drag-and-drop interface that accelerates development and reduces the need for extensive coding. This approach enables quicker time-to-market by offering reusable components for the entire ML lifecycle, from data handling to algorithm deployment. These platforms also simplify model maintenance through continuous monitoring and automated retraining, ensuring models remain accurate and effective. Moreover, low-code tools help bridge the talent gap by allowing non-experts to participate in ML development, reducing the dependency on scarce data science talent.
Case Study: United States Cold Storage
United States Cold Storage (USCS) leveraged low-code ML platforms to develop an automated appointment scheduler, resulting in a 16% reduction in warehouse turn times and $300K in savings within a quarter. The low-code approach enabled rapid development and scaling of the solution across multiple locations, highlighting its potential to lower the total cost of ownership for AI initiatives.
Low-code ML platforms are thus a valuable tool for organizations looking to accelerate AI adoption and maximize business impact.
Top Low-Code Machine Learning Platforms for Beginners
1. PyCaret
Overview: An open-source machine-learning library in Python that simplifies creating and deploying ML models. It acts as a wrapper over multiple ML libraries like scikit-learn and XGBoost, reducing coding requirements.
Key Features: Speedy development, minimal coding, supports various ML libraries.
2. Auto-ViML
Overview: A tool that quickly builds ML models by automatically rendering data through different models and selecting the best one. It also preprocesses data automatically.
Key Features: Automated data cleaning, transformation, and normalization; works with various data types.
3. H2O AutoML
Overview: An open-source ML platform known for its AutoML feature, which automates the process of building and testing multiple ML models simultaneously.
Key Features: Supports widely used ML algorithms, beginner-friendly, automates model building.
4. Google Cloud AutoML
Overview: A no-code tool that enables anyone to train and deploy custom ML models. It supports various data types and covers a wide range of use cases.
Key Features: Facilitated data labeling, supports computer vision and NLP, scalable to Google Cloud.
5. Google ML Kit
Overview: A toolkit for Android and iOS developers that provides APIs for implementing ML features like bar scanning and face detection without extensive coding.
Key Features: Real-time processing on mobile devices, beginner-friendly API integration.
How did you like today's email? |
If you are interested in contributing to the newsletter, respond to this email. We are looking for contributions from you — our readers to keep the community alive and going.