- Data Pragmatist
- Posts
- Descriptive Statistics, ChatGPT prompts to improve your writing
Descriptive Statistics, ChatGPT prompts to improve your writing
A set of methods and techniques used to describe and summarize data
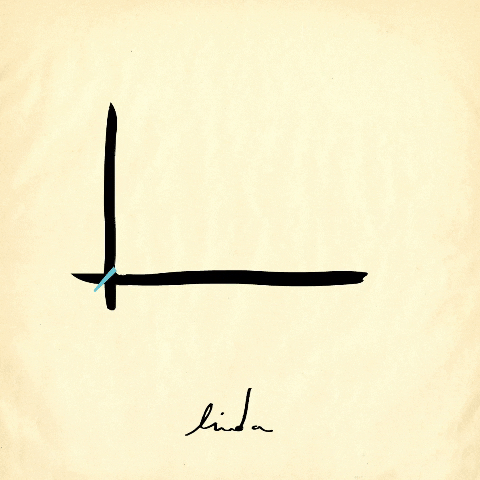

Welcome to learning Wednesday edition of the Data Pragmatist, your dose of all things data science and AI.
π Estimated Reading Time: 4 minutes. Missed our previous editions?
Today we are talking about a set of methods and techniques used to describe and summarize data. As part of our learning series, ChatGPT prompts to improve your writing.
β Arun Chinnachamy
π12 ChatGPT Prompts to Take Your writing to the Next Level
Blog Research and Planning
Suggest [X] topic ideas for a blog post on [subject]. Include the primary keyword [X] in all the topics
Generate a blog post outline on the topic [X]. The outline must have [Y number of] subheadings
Suggest some H2 and H3 subheadings for the content given below. Donβt modify the text.
Generate an SEO title and a meta description for a blog post on the topic [Topic], and focus keyword [keyword]
Blog Writing
Write a blog post introduction on [Topic]. Write it in a [X] tone. Use transition words. Write over [Y] words. Include the following keywords:
Write an introduction for the section [section name/subheading] of a blog post on [Topic]
What are the main takeaways that a reader should be left with after reading a blog on [topic]
You are a [tech writer/news writer/creative writer/business writer/lifestyle writer]. You have to write an engaging [review/listicle/how-to-guide/case study/news story/opinion piece] on the topic [Topic]. I will provide you with the basic points that need to be covered. You can ask for screenshots to be included in different parts of the text, and I will add them later. Here is what you need to cover
Blog Optimization
Rewrite the following paragraph in a [conversational, assertive, humorous, etc.] tone
Check the following article for redundant words/sentences and rewrite where necessary:
Rephrase this content in [X] ways. Retain the original meaning and avoid repetition of points.
Recommendation Creator Science Become a smarter creator in just 10 minutes per week. | |
Subscribe |
π§ Featured Concept: Descriptive Statistics
Data analysis plays a pivotal role in various fields, from science and business to social sciences and healthcare. Regardless of the size and complexity of the datasets, statistics serve as a crucial tool to extract meaningful insights from data. Descriptive statistics, in particular, offer a fundamental framework for understanding and presenting data in a comprehensible and insightful manner.
What is Descriptive Statistics?
Descriptive statistics encompass a set of methods and techniques used to describe and summarize data. These methods help us in presenting data in a meaningful way that can be easily understood. This aspect of statistics is particularly valuable when working with small datasets. However, the insights gained from descriptive statistics can also be instrumental in predicting future trends based on current findings. The primary goal of descriptive statistics is to provide a clear and concise representation of data, making it an essential tool for data analysts.
Types of Descriptive Statistics
Descriptive statistics can be categorized into three primary types, each serving a unique purpose:
Measures of Central Tendency
Measure of Variability
Measures of Frequency Distribution
Measures of Central Tendency
Measures of central tendency are used to represent the central or typical values in a dataset. These measures provide insights into where the data clusters. The three main measures of central tendency are:
Mean: The mean is the average of all data points and is calculated by summing all observations and dividing by the number of data points.
Mode: The mode is the value that occurs with the highest frequency in the dataset. A dataset may have no mode if all data points have the same frequency.
Median: The median is the middle value of the dataset. It separates the data into two equal halves. If the dataset has an odd number of elements, the median is the center value, while in even datasets, it's the average of the two central elements.
Let's consider a Python example to calculate the mean:

This code would output: "Mean = 7.333333333333333."
Measure of Variability
Measures of variability, also known as measures of dispersion, provide insights into how the data is spread or dispersed. Key measures of variability include:
Range: The range is the difference between the largest and smallest data points. A larger range indicates a more spread-out dataset.
Variance: Variance is an average of squared differences between each data point and the mean. It quantifies the overall spread of data points.
Standard Deviation: The standard deviation is the square root of the variance. It quantifies the average distance of data points from the mean.
To illustrate, here is a Python code example to find the variance:

This code would produce the output: "Var = 2.5."
Measures of Frequency Distribution
Measures of frequency distribution provide valuable insights into how data is distributed and its characteristics. These measures include:
Count: Counting the occurrences of values in a dataset.
Frequency: Determining how often specific values appear in the dataset.
Relative Frequency: Expressing frequencies as proportions or percentages.
Cumulative Frequency: Summing frequencies to create cumulative distribution.
Univariate vs. Bivariate Analysis
Univariate Analysis:
Single Variable Focus: Univariate analysis centers on examining a single variable or data attribute at a time.
Purpose: Its primary purpose is to gain insights and understanding about the characteristics and behavior of that individual variable.
Data Exploration: Univariate analysis involves summarizing and visualizing data for a single variable without considering its relationship with other variables.
Common Techniques: Histograms, box plots, summary statistics, and frequency distributions are common tools used in univariate analysis.
Bivariate Analysis:
Dual Variable Examination: Bivariate analysis involves the simultaneous examination of two variables to understand their relationship or interaction.
Purpose: It aims to explore how two variables are connected and whether there is any correlation, causation, or dependency between them.
Data Interaction: Bivariate analysis considers how changes in one variable relate to changes in another, helping in making informed decisions based on these relationships.
Common Techniques: Scatter plots, correlation coefficients, covariance, and cross-tabulation are techniques often employed in bivariate analysis.
Decision-Making: Bivariate analysis assists in making decisions that involve one variable influencing or being influenced by another, enabling better strategies and predictions.
Descriptive statistics provide a foundational framework for data analysts to explore and understand datasets. By revealing central tendencies, variations, and frequency distributions, descriptive statistics make data more interpretable and insightful. Whether in scientific research, business decision-making, or social sciences, the power of descriptive statistics is undeniable in uncovering the hidden treasures within data.
How did you like today's email? |
If you are interested in contributing to the newsletter, respond to this email. We are looking for contributions from you β our readers to keep the community alive and going.