- Data Pragmatist
- Posts
- Exploring Time-to-Event with Survival Analysis
Exploring Time-to-Event with Survival Analysis
Mistral's latest model sets new records for open source LLMs

Welcome to learning edition of the Data Pragmatist, your dose of all things data science and AI.
π Estimated Reading Time: 5 minutes. Missed our previous editions?
β Arun Chinnachamy
π12 ChatGPT Prompts to Take Your writing to the Next Level
Blog Research and Planning
Suggest [X] topic ideas for a blog post on [subject]. Include the primary keyword [X] in all the topics
Generate a blog post outline on the topic [X]. The outline must have [Y number of] subheadings
Suggest some H2 and H3 subheadings for the content given below. Donβt modify the text.
Generate an SEO title and a meta description for a blog post on the topic [Topic], and focus keyword [keyword]
Blog Writing
Write a blog post introduction on [Topic]. Write it in a [X] tone. Use transition words. Write over [Y] words. Include the following keywords:
Write an introduction for the section [section name/subheading] of a blog post on [Topic]
What are the main takeaways that a reader should be left with after reading a blog on [topic]
Blog Optimization
Rewrite the following paragraph in a [conversational, assertive, humorous, etc.] tone
Check the following article for redundant words/sentences and rewrite where necessary:
Rephrase this content in [X] ways. Retain the original meaning and avoid repetition of points.
π§ Exploring Time-to-Event with Survival Analysis
Survival analysis is a statistical method used to analyze the time until an event of interest occurs. Initially developed in the medical field, it has found applications in diverse domains like predictive maintenance, customer analytics, and loan modeling.
Key Concepts
Survival analysis revolves around defining the event of interest and the survival duration related to it.
Event: It refers to an unambiguous and binary occurrence, such as the death of a biological entity or machinery failure.
Lifeline/Survival Duration: This indicates the time until the event occurs or until the observation ends.
Applications
Survival analysis finds applications in various scenarios, including:
Modeling User Behavior: Predicting user conversion to membership or purchase.
Predictive Maintenance: Estimating time until machine failure.
Healthcare: Assessing the likelihood of cancer recurrence.
Human Resources: Predicting the time until employee turnover.
Survival and Hazard Functions
The survival function S(t) represents the probability of an object's survival beyond time t, while the hazard function h(t) indicates the probability of an event occurring at time t, given survival up to that point.

Survival function equation

Hazard function equation
Dataset Considerations
Survival analysis requires datasets containing individual observations of events, including event occurrence (binary) and duration of observation. Censorship, where survival duration is partially known, must also be considered, with right censorship being the most common type.
Survival Analysis Techniques
Several techniques are employed in survival analysis:
Kaplan-Meier Estimator: Non-parametric method for estimating the survival function.
Log-rank Test: Compares survival times between groups.
Cox Proportional Hazards Model: Describes the effect of variables on survival.
Kaplan-Meier Estimator
The Kaplan-Meier estimator is particularly useful for modeling survival without assuming a specific underlying distribution. It estimates the survival function based on observed data.
In this model, the Survival function S(t) is estimated with the below formula.

The Kaplan-Meier estimator
Weibull Model
The Weibull model allows for the analysis of multiple variables on survival functions. It assumes survival times follow a Weibull distribution, providing a continuous probability distribution for survival.
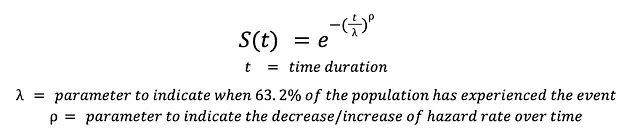
Survival function on Weibull model
Cox Proportional Hazards Model
The Cox PH model evaluates the effect of different factors on survival, assuming proportional hazards and no interactions among variables.
Survival analysis offers valuable insights into the expected duration until an event occurs. While initially developed for medical research, its applications extend to various domains. Understanding survival and hazard functions, selecting appropriate techniques like the Kaplan-Meier estimator and Cox PH model, and interpreting results are crucial for effective analysis across different scenarios.
π Meta adds AI to its Ray-Ban smart glasses LINK
Ray-Ban Meta smart glasses now include multimodal AI, enabling the device to process diverse types of data such as images, videos, text, and sound to understand the userβs environment in real-time.
The AI capabilities allow users to interact with their surroundings in enhanced ways, such as identifying dog breeds, translating signs in foreign languages, and offering recipe suggestions based on visible ingredients.
Initial testing of the multimodal AI has shown promise, although it has also revealed some inconsistencies in accuracy, such as errors in identifying certain car models and plant species.
π Mistral's latest model sets new records for open source LLMs LINK
French AI startup Mistral AI has released Mixtral 8x22B, claiming it to be the highest-performing and most efficient open-source language model, utilizing a sparse mixture-of-experts model with 39 billion of its 141 billion parameters active.
Mixtral 8x22B excels in multilingual support and possesses strong math and programming capabilities, despite having a smaller context window compared to leading commercial models like GPT-4 or Claude 3.
The model, licensed under the Apache 2.0 license for unrestricted use, achieves top results on various comprehension and logic benchmarks and outperforms other models in its supported languages on specific tests.
How did you like today's email? |
If you are interested in contributing to the newsletter, respond to this email. We are looking for contributions from you β our readers to keep the community alive and going.